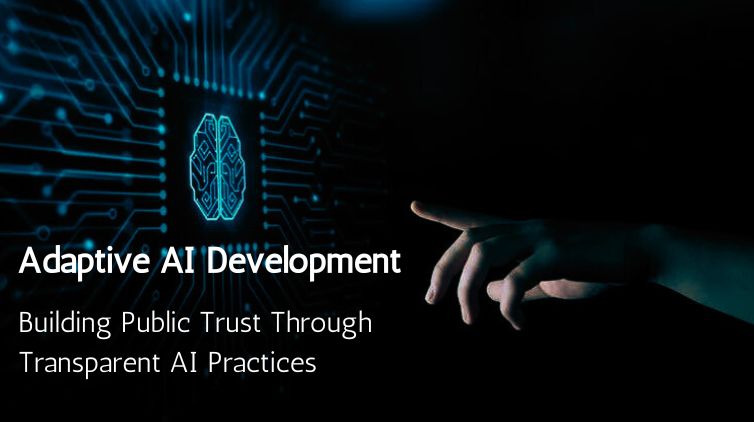
As artificial intelligence advances, maintaining public trust will require responsible transparent practices that give people meaningful visibility into and influence over AI systems. Let us explore the various dimensions of transparency and how the AI community can nurture informed trust.
Table of Contents
Why AI Transparency Matters
Being transparent and opening up AI systems to oversight serves many important purposes for society. Lawmakers need to understand how AI systems work, what they are capable of, and their limitations. This allows the creation of balanced guardrails and rules through sound regulation and governance.
Explanations and access enable smarter policies that protect the public while supporting innovation. Transparency helps set realistic public expectations about AI capabilities. Hype and fearmongering thrive when systems are opaque black boxes. Thoughtful transparency separates fact from fiction. It grounds public discourse in reality rather than hyperbole. The public deserves accurate mental models of existing technology.
Giving the public, especially groups impacted by AI, a meaningful voice in shaping these technologies builds legitimacy and trust. Participatory design makes people feel empowered over changes affecting their lives. The collaboration demonstrates respect and ensures local interests are considered. Shared influence cultivates buy-in through active listening.
Transparency can surface potential risks or harms early on for correction before real-world damage occurs. Rigorous testing and auditing by unbiased experts safeguard the public interest. Identifying issues while still on the drawing board prevents avoidable disasters once deployed at scale. Care and accountability avert crises.
If issues do emerge post-launch, transparency provides accountability. Independent monitoring and citizen reporting channels create checks on power to uphold justice. Openness enables course correction. Introspection improves when shortcomings see light. Encouraging beneficial learning between AI developers and society is also key. Understanding each other’s perspectives and values helps steer progress responsibly.
More minds enlighten the path forward together. Cross-disciplinary exchange grounds technology in shared human needs and ethics. Ultimately, transparency demonstrates good faith efforts to act in the public’s interest. Openness breeds trust in intentions and competence. Secrecy sows doubt and suspicion. Responsible innovation should move boldly but cautiously, with light to guide rather than cover its actions.
Of course, full transparency has limits. Commercial protection of intellectual property remains reasonable for funding innovation. Security considerations also warrant discretion regarding potential misuse. Complete openness cannot be expected. But darkness breeds mistrust. With care and wisdom, transparency practices can strike an optimal balance that convenes common cause between AI creators and the broader public. Our shared future is brightened when illuminated together through respectful engagement.
Dimensions of AI Transparency
Useful frameworks distinguish several important dimensions of transparency:
Development Transparency
Sharing design choices, training data, testing methods, and capabilities. Explains how AI works and is built responsibly.
Application Transparency
Communicating where and how AI is deployed and its real-world impacts. Contextualizes uses.
Organizational Transparency
Opening governance, ethics reviews, and security practices to oversight. Conveys institutional priority on responsibility.
Interactive Transparency
Enabling public experimentation with models to intuit behaviors through experience. Builds intuitive understandings.
Balancing these facets supports informed trust in the societal role of AI.
Current Approaches to Transparent AI
Adaptive AI Development Company employs various practices to enhance AI transparency:
Explainability Methods
Tools that help describe AI reasoning behind outputs in plain terms, such as:
- Local linear approximations explaining models in generalized ways people understand
- Visualizations depicting how algorithms operate on data flows
- Natural language interfaces elucidating logic behind conclusions
Documentation Standards
Careful documentation explaining:
- Training data characteristics, cleaning processes, and potential limitations
- Testing methodology, benchmarks, and performance results
- Model architectures, equations, and key parameters
- Version history tracking incremental changes
Provenance Tracking
Version control and metadata standards that:
- Log model iterations from inception to deployment
- Watermark production systems for monitoring unauthorized copying
- Label data sources and processing provenance
Participatory Design
Involving external stakeholders directly in development, via:
- Focus groups giving feedback on product concepts and options
- Beta testing periods gather user experiences before the launch
- Community advisory boards weighing in on plans and policies
- Public debates on ethical considerations and oversight mechanisms
Lifecycle Oversight
External reviews assessing AI responsibility:
- Pre-launch audits by independent panels flagging risks
- Post-launch performance monitoring by third parties checking for harms
- Citizen grievance redress mechanisms to report issues
This interdisciplinary collaboration strengthens responsible innovation.
Key Challenges around Transparency
While crucial, transparency also involves valid tensions we must thoughtfully navigate:
- Commercialization – Businesses must balance transparency with reasonable IP protections and competitive practices.
- Gaming – Bad actors could exploit details shared for harmful deception. Security discretion is warranted.
- Misconceptions – Laypeople may draw faulty conclusions from transparency efforts without proper context. Careful communication is essential.
- Engagement Inequities – Participatory initiatives could over-represent certain voices without proactive inclusion. Varied outreach is vital.
- Slowing Innovation – Oversight processes add friction that potentially delays beneficial advances if not managed judiciously. Prioritization prudence helps.
With care and wisdom, transparency can be implemented in ways that maximize public benefit while minimizing risks. But we must be vigilant.
Providing Visibility into Data Practices
Transparency around AI training data is crucial for establishing trust:
- Documenting dataset sources, collection methods, cleaning processes, and benchmark results. Provenance supports accountability.
- Performing bias audits by respected third parties to certify appropriateness for intended contexts. Independent assessment reassures.
- Enabling review boards and community representatives to inspect data samples used in high-stakes systems. Direct visibility deters abuse.
- Watermarking or fingerprinting datasets to trace lineage and combat piracy. Attribution maintains integrity.
- Expiring and right-to-use limitations on datasets built from individual contributions. Respect autonomy and consent.
- Secure multiparty computation techniques that train models without exposing raw data. Maintain privacy while enabling oversight.
- Differential privacy and other mathematical noise techniques allow general insights from data while protecting individual identities. Preserve anonymity.
- Published data sheets detailing composition, collection methods, annotation processes, and suitable use cases. Inform sound application.
- Responsible data practices provide the foundation on which trust in AI systems must be built.
Explaining How AI Systems Operate
People want to understand how AI arrives at outputs. Explainability methods help:
- Interactive visualizations demonstrate how data flows through algorithms to yield conclusions. Animated workflows build intuition.
- Local approximation explanations show the most influential factors behind specific model predictions. Demystify individual results.
- Counterfactual testing shows how tweaking inputs alters outputs to reverse-engineer relationships. Hands-on inference calibration.
- Sensitivity analysis quantifies how much perturbing different parameters impact outcomes. Reveals internal logic.
- Natural language narratives of model reasoning are rendered in plain terms. Humanize opaque calculations.
- Representation learning techniques force models to structure and explain knowledge more like people. Anthropomorphic reasoning aids comprehension.
- Quantified measures of explanation confidence when uncertainties exist. Convey limits openly.
- Explanations adapted to different audience expertise levels. Make technical concepts accessible while preserving nuance.
- Clear explanations enable sound oversight by illuminating how AI interprets the world.
Detailing How AI Improves Over Time
Version histories tracking incremental model changes support monitoring improvement:
- Comprehensive logs recording all experiments, iterations, parameters, and performance metrics over development.
- Model cards published alongside research papers summarizing key technical details for replicability and appropriate application. Universally expected documentation.
- Changelog conventions detailing the motivation and impacts of each update. Guide oversight.
- Before/after testing quantifying effects of changes on performance, fairness, robustness and other criteria. Validate progress.
- Version labelling embedded in production systems enables the tracing of current and past states. Maintain traceability.
- Maturity assessments benchmarking capabilities against human performance. Convey current limitations accurately.
- Forward-looking roadmaps outlining priorities for future self-improvement. Set realistic expectations about the pace and challenges ahead.
- Continuous monitored improvement provides confidence in responsibly expanding empowerment.
Enabling Responsible Public Experimentation
Providing interactive access to AI systems in controlled ways can aid public understanding and build trust:
Carefully scoped online sandboxes could allow people to tweak parameters on certain AI models and directly observe resulting behaviours.
This facilitates inquisitiveness through hands-on tinkering.
Step-by-step tutorials could introduce key capabilities and limitations of AI systems through guided exploration. This structures learning in an accessible way.
Educational lesson plans could harness interactive AI models to teach students principles of machine learning, statistics, ethics and more.
This inspires future generations through technology literacy.
Public contest platforms could let researchers and enthusiasts constructively try to ‘break’ systems by creatively testing vulnerabilities.
This crowd sourced adversarial collaboration strengthens system resilience.
Model zoos could curate a catalogue of interactive systems for the public to safely inform and delight. This showcases innovation responsibly.
Strict terms of use must prohibit malicious actors from exploiting interfaces. This maintains security while enabling controlled access.
Usage should be monitored to catch any emerging risks early.
Parameters and capabilities granted should be gradually expanded based on evidence of responsible engagement.
Under proper governance, enabling public interaction and tinkering could cultivate sound intuitions and foster informed, balanced perspectives on AI behaviours and limitations.
Structuring AI Oversight Responsibly
Independent oversight provides expert assurance while avoiding obstruction:
- Multidisciplinary review boards with rotating membership representing diverse constituencies like academia, engineering, philosophy, law, and users. Counter insularity.
- Published standards of practice that boards use to evaluate systems under review. Codify responsible innovation principles.
- Multi-stage engagement processes allow iterative refinement in response to board feedback before launch. Incorporate wisdom early.
- Exception handling policies that expedite approval in cases of urgent need, while maintaining review integrity. Balance diligence with agility.
- Secure facilities allowing hands-on inspection of systems by review boards under non-disclosure agreements. Enable depth of evaluation.
- Post-launch performance monitoring with watchdog authority to recommend pausing deployed systems if harm is detected, subject to checks and balances. Responsiveness without overreach.
- Carefully structured oversight harnesses diverse expertise to guide beneficial innovation while avoiding obstructionism. Getting governance right is key.
Nurturing a Culture of Ethics
Instilling strong ethical values strengthens accountability:
- Codes of ethics openly define expected principles and duties. Set clear expectations at all levels
- Ethics training developed in collaboration with civil society groups. Move beyond compliance thinking to integrate ethics intrinsically.
- Incentive structures rewarding responsible innovation focused on collective well-being over purely financial motives. Compensate conscience appropriately.
- Courageous leadership that confronts and resolves tensions between principles and business incentives. Model integrity from the top.
- Whistleblower policies protect those reporting violations from retaliation. Safeguard truth-tellers.
- Participatory team ceremonies reflect on ethical duties and how to uphold them in daily work. Ritualize wisdom.
- A shared commitment to moral courage and truth-seeking guides organizations toward the light.
Establishing Robust Cybersecurity
Protecting transparent AI from misuse requires strong cyber security:
- Encryption for data storage and transfer. Prevent unauthorized access to sensitive training data or machine learning models.
- Access controls restrict interface usage only to authorized parties. Maintain the integrity of oversight mechanisms.
- Continuous vulnerability testing by white hat hackers to probe systems proactively. Identify and fix weaknesses before exploitation.
- Monitoring systems for suspicious activity indicating potential intrusions. Rapid response capabilities help contain threats.
- Compartmentalization isolates components to limit damage if any one part is breached. Avoid single points of failure.
- Resilience engineering to ensure critical functions degrade gracefully even when
attacked. Fail-safe, not dangerously.
- Cybersecurity certification requiring external audits validating defenses meet industry best practices. Accountability drives continual improvement.
- Staff training on secure protocols and recognizing threats. Savvy employees provide another layer of protection.
With expanding transparency comes responsibility to prevent harm from those with ill intent. Diligent cyber security enables the safe sharing of innovations.
Conveying Appropriate Context
Transparency without context risks of project Q-Star misinterpretations. Responsible framing is key:
Accessible explanations of the science to correctly convey capabilities and limitations. Prevent both hype and unjustified fear.
Histories of incremental development are required. Managing expectations prevents disappointment.
Comparisons against rigorous benchmarks provide a grounded sense of strengths and weaknesses. Illuminate gaps requiring improvement.
Cautious language hedging limitations and uncertainties that still exist. Measured communication avoids absolutist thinking.
Model cards and nutrition labels cataloguing performance dimensions prevent cherry-picking or decontextualized critique.
Diverse perspectives included to counter tendencies toward insular groupthink. Incorporate broad viewpoints.
Respect for public intelligence and values. Condescension breeds mistrust. Frank discourse unifies.
With care and wisdom, transparency fortifies progress through constructive collaborative engagement.
With comprehensive transparency across tools, data, oversight and culture, the path ahead can be one of collaborative trust-building that realizes AI’s benefits responsibly.
The future remains open – let us approach it together openly.
With creativity and good faith efforts, Adaptive AI Development Company can forge a vibrant culture of transparency and trust that benefits all of society. The path forward requires working together.
What other ideas do you have for improving public transparency around AI to build trust? We are excited to brainstorm further.